“Data is the pollution problem of the information age, and protecting privacy is the environmental challenge.” – Bruce Schneier
Today it is difficult to do just about anything online without a company, or two or more knowing who you are, where you are, and where you have been. We live in a time where digital surveillance has never been more pervasive. Analytics are running constantly and opaquely behind the scenes to learn everything about you for reasons such as predicting your next move, what to sell to you, or how best to sell your data to others.
At Anonyome Labs, we are providing protection for the online community with the MySudo application and Sudo Platform. MySudo is an easy to use tool for creating online identities equipped with a customizable name, phone number, email address, private browser and virtual cards. Sudo Platform allows enterprises to embed the privacy technologies showcased in MySudo into their own applications.
As a data scientist, I was trained to want any and all data to throw into a model and become a prediction hero. As a privacy advocate, I acknowledge that analytics cannot be the wild west, and gladly accept the challenge to help inform Anonyome’s business without becoming what we are helping users to protect against. In the rest of this article, I will discuss how analytics for MySudo is operated under a privacy lens.
From the perspective of the privacy conscious consumer, including the Anonyome team who identify as such, “less is best” when it comes to processing personal data. This starts with minimizing data collection. We do not collect your legal identity information or other personal data before you start using our services, with the exception of regulated parts of our offering such as virtual cards. You can read more about this in this FAQ. If we don’t collect your personal data, we can’t use it, misuse it or lose it if we were to experience a data breach (and we work hard to make sure that doesn’t happen). The “less is best” core value aligns well to minimizing dark data, a form of data debt accrued over time. Gartner defines dark data as “Information assets organizations collect, process, and store during regular business activities, but generally fail to use for other purposes” [1]. Dark data will remain at a minimum by Anonyome’s choice to intentionally store less information about our consumers.
User content, such as the text of email messages, SMS messages and the like, is never processed beyond what you would expect us to do – deliver the messages to your intended recipients or to you in the most secure way possible. If the communications are from MySudo user to MySudo user, they’re end-to-end encrypted and Anonyome can’t read the messages even if we wanted to (we don’t). For messages sent or received via regular SMS or email, they may be visible to third parties when they pass through networks beyond the control of the Sudo Platform. Read more in this FAQ article.When we analyze the usage of MySudo across our user base, we’re usually interested in aggregated time series data. For example, how many calls and messages were made last week across all MySudo users, is the relationship between ratio of incoming to outgoing email messages changing over time across all MySudo users? This analysis does not require long term access to the content or metadata of individual user communications, so we don’t preserve that beyond what is required for our users to use MySudo and access their messages and call logs. An example of how this aggregate works in practice is illustrated in the diagram below.

Some usage requires that uniqueness be preserved, without needing to know the value that makes the data unique. For example, to analyze how users are consuming their subscribed entitlements under a paid MySudo subscription, we need to be able to differentiate between the activity of different MySudo phone numbers, without needing to know what the specific phone numbers are. Tokenization is a form of de-identification and cryptographic hashing is a useful technique for doing so. Hashing provides protection by converting input to an output that appears random but maintains uniqueness. In this way, analytics can be performed on the data without needing to know actual identity values. When implemented well, it is also computationally very difficult to convert back to its original form.
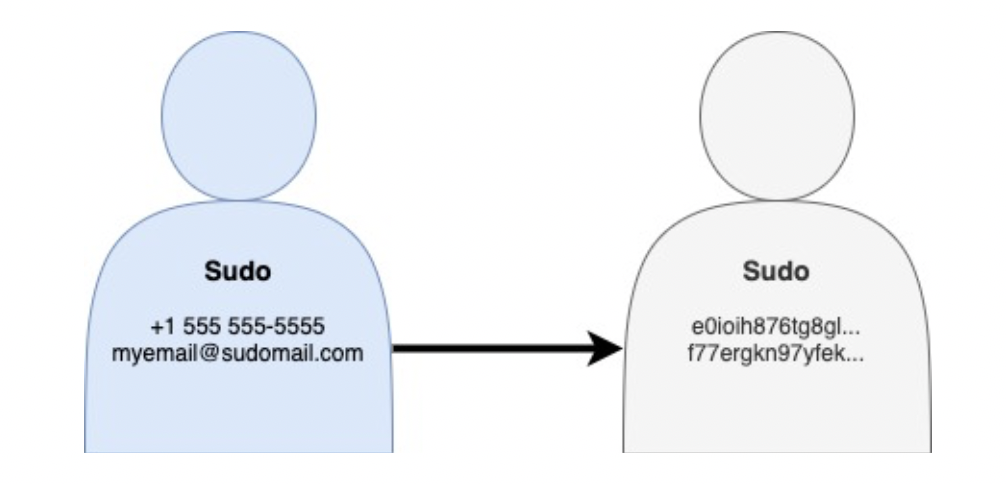
A second technique is to reduce the precision or accuracy of data. For this, we use techniques such as differential privacy, where we introduce randomly generated noise to the data and rounding of numerical data elements. This reduces the re-identification risk of the data while preserving a reduced but acceptable level of analysis against our business objectives.
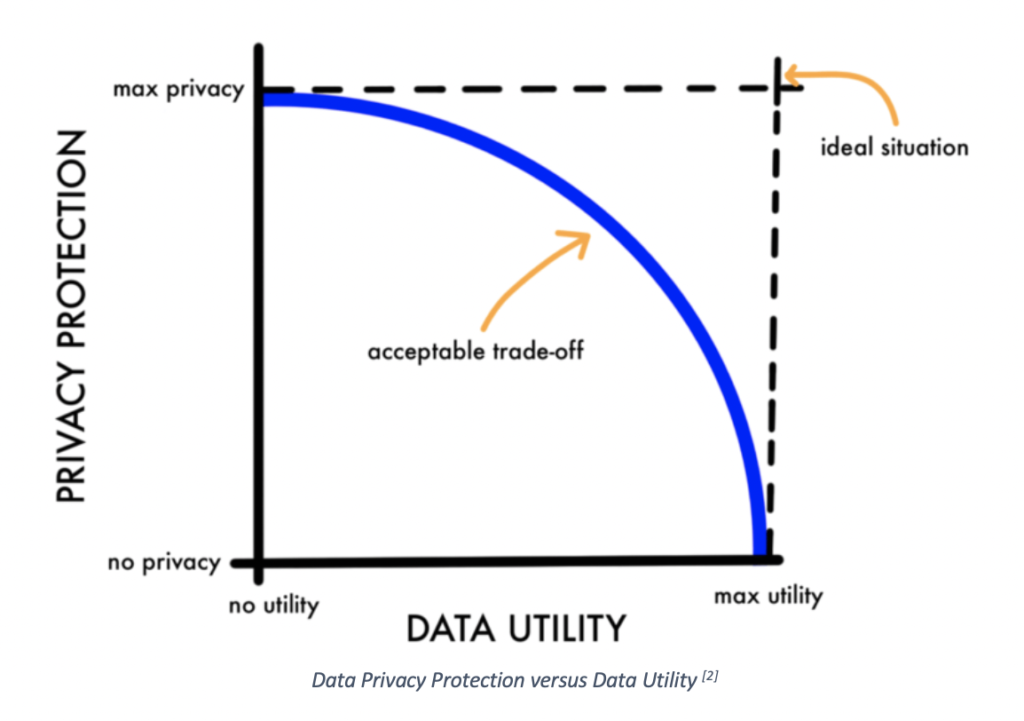

Whether the data is aggregated or individual, we define explicit retention periods for the data we hold. You can read more about what we do with MySudo in this FAQ article. By contrast, we see the choice of others to keep data “indefinitely” as rarely a thoughtful choice and more likely associated with mindsets of “don’t know why we even have this data” and “it’s not important to us to think about consumer privacy until we are forced to”. I believe this thinking is now antiquated.
Lastly, as described in our privacy policy, we never sell the limited data we do have to third parties, and we never will.
I’d like to think that Anonyome is a leader in defining a more responsible way to think about, implement and use analytics. I hope this article has given you some ideas on how to adopt a more privacy sensitive approach to analytics in your own organization. Your users will thank you for it!
References:
[1] Gartner_Inc. (n.d.). Dark Data. Retrieved from https://www.gartner.com/en/information-technology/glossary/dark-data
[2] Nelson, Gregory. (2015). Practical Implications of Sharing Data: A Primer on Data Privacy, Anonymization, and De-Identification.
Image Source: https://unsplash.com/photos/d9ILr-dbEdg